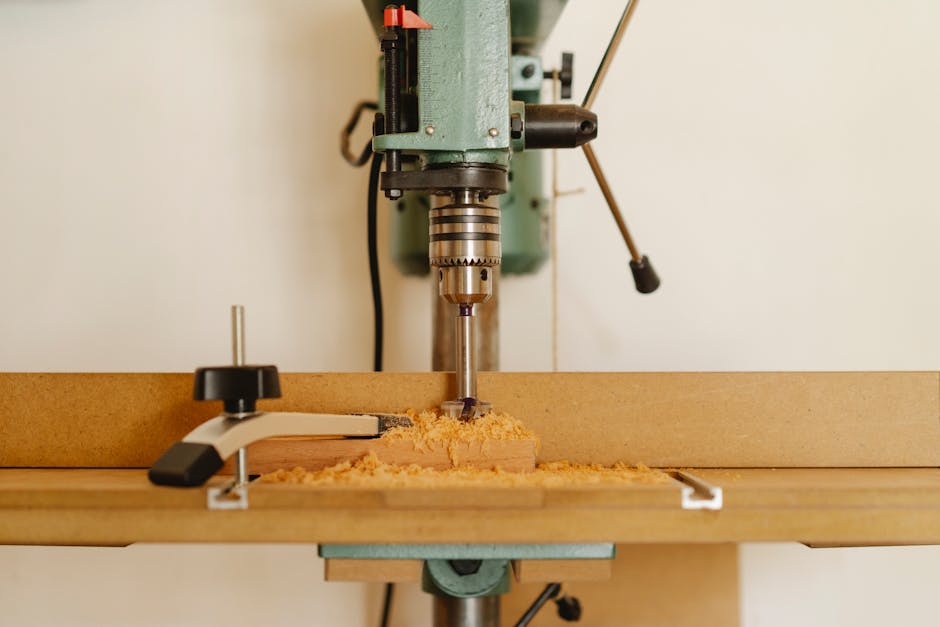
Machine Learning in Predictive Analytics
Machine Learning in Predictive Analytics
Predictive analytics is a powerful technique that leverages historical data to make accurate predictions about future outcomes. It has revolutionized various industries, from healthcare to finance to marketing. One of the key components of predictive analytics is machine learning, a subset of artificial intelligence that enables systems to learn from data and improve their performance over time.
Machine learning algorithms analyze large datasets and identify patterns and relationships. These algorithms can then apply what they have learned to new, unseen data, making predictions or decisions with minimal human intervention. This ability to learn and adapt is what makes machine learning so valuable in predictive analytics.
Machine learning in predictive analytics has numerous applications. For example, in the financial industry, machine learning algorithms can analyze historical trading data to predict stock prices or identify potential fraud. In healthcare, machine learning models can analyze patient data to predict disease progression or recommend personalized treatments. In marketing, machine learning can help target specific customer segments, optimize pricing strategies, and personalize content recommendations.
There are different types of machine learning algorithms used in predictive analytics, including supervised learning, unsupervised learning, and reinforcement learning. Supervised learning involves training a model with labeled data, where the desired outcome is known. Unsupervised learning, on the other hand, involves discovering patterns or relationships in unlabeled data. Reinforcement learning involves training an agent to take actions in an environment to maximize rewards.
As with any data-driven approach, machine learning in predictive analytics requires careful data preparation, feature selection, and model evaluation. It's important to ensure the quality, relevance, and accuracy of the data used for training the machine learning models. Additionally, model performance should be regularly monitored and evaluated to identify potential issues and improve accuracy.
Machine learning continues to evolve, and its integration with predictive analytics holds great promise for improving decision-making and driving innovation in various fields. By leveraging historical data and applying advanced algorithms, organizations can gain valuable insights, make predictions, and optimize their strategies for better outcomes.