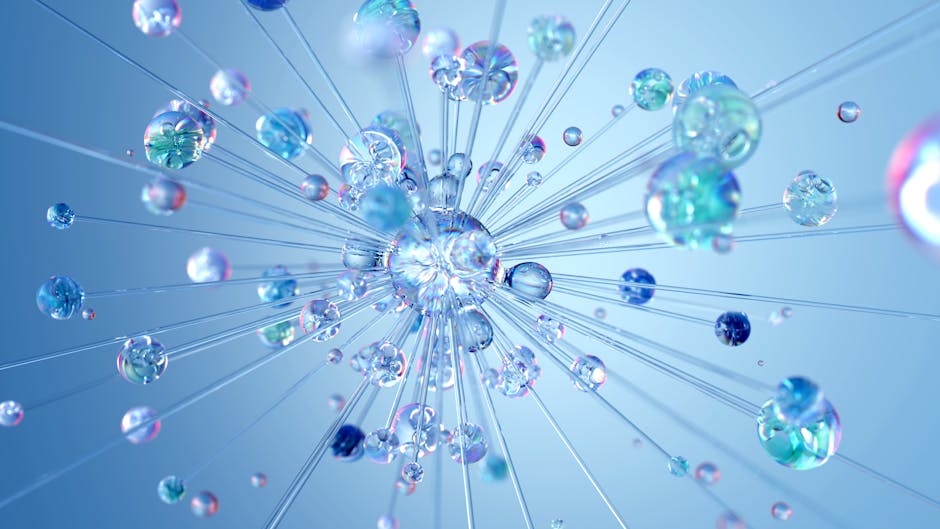
Machine Learning for Credit Scoring
Machine Learning for Credit Scoring
In recent years, machine learning has become an integral part of the lending industry. The traditional approach to credit scoring, which relied heavily on historical data and predefined rules, is now being replaced by more sophisticated AI algorithms.
One of the main advantages of using machine learning for credit scoring is its ability to analyze a vast amount of data from various sources. Instead of relying solely on credit history, machine learning models can incorporate alternative data points, such as social media activity, online shopping behavior, and even smartphone usage patterns.
By considering these additional factors, machine learning algorithms can provide a more accurate assessment of an individual's creditworthiness, especially for those with limited credit history or unconventional financial profiles.
Another benefit of using machine learning for credit scoring is its adaptability. Traditional scoring models often struggle to keep pace with rapidly changing economic conditions and emerging trends in consumer behavior. On the other hand, machine learning models can quickly adapt to new patterns and adjust their predictions accordingly.
However, implementing machine learning in credit scoring is not without its challenges. One of the main concerns is the potential for bias in AI algorithms. If trained on biased historical data, machine learning models can perpetuate existing inequalities in lending decisions.
Moreover, machine learning models can be complex and lack transparency, making it difficult to interpret how they arrived at a particular credit score. This lack of explainability raises ethical concerns and can make it challenging for consumers to understand and contest lending decisions.
Despite these challenges, the adoption of machine learning in credit scoring is steadily increasing. The lending industry recognizes the potential of AI algorithms to improve accuracy, speed, and efficiency in determining creditworthiness.
As machine learning continues to evolve, it is crucial to address the ethical and regulatory implications associated with its use in credit scoring. Striking the right balance between innovation and fairness will be essential in ensuring that machine learning algorithms work in the best interest of both lenders and borrowers.