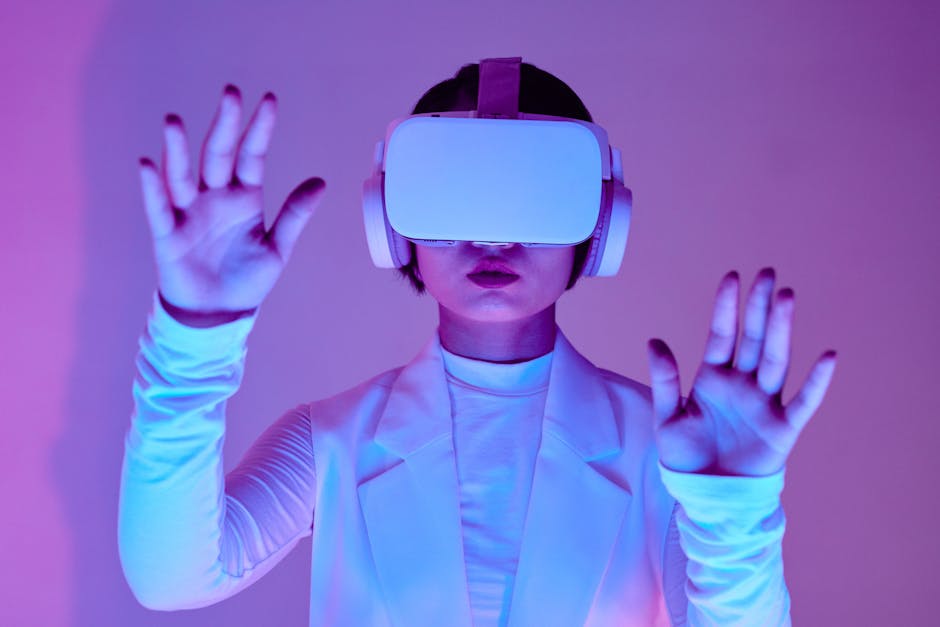
Interdisciplinary Approaches in AI Research
Interdisciplinary Approaches in AI Research
Artificial Intelligence (AI) has become one of the most promising fields in technology, with applications ranging from self-driving cars to virtual assistants. However, AI research is not limited to computer science alone. Interdisciplinary approaches that combine diverse fields and expertise have proven to be highly effective in pushing the boundaries of AI capabilities.
One key benefit of interdisciplinary approaches in AI research is the ability to tackle complex problems from multiple angles. By bringing together experts from different domains such as mathematics, psychology, and biology, researchers can gain a more comprehensive understanding of AI systems and their impact on society.
For instance, cognitive psychology plays a crucial role in understanding human learning and decision-making processes. By incorporating psychological principles into AI algorithms, researchers can improve the efficiency and accuracy of AI systems, leading to better performance in tasks like image recognition or natural language processing.
Another example of interdisciplinary collaboration in AI research is the combination of computer science and linguistics. By leveraging linguistic theories and knowledge, researchers can develop AI models that understand and generate human language more effectively. This can have significant implications in various areas such as machine translation, sentiment analysis, and dialogue systems.
Furthermore, interdisciplinary approaches in AI research facilitate innovation by bringing together diverse perspectives. Collaborations between engineers, designers, and social scientists can result in AI systems that are not only technologically advanced but also user-friendly, ethical, and socially responsible.
In conclusion, interdisciplinary approaches have become a driving force in AI research. By integrating knowledge and expertise from multiple fields, researchers can overcome complex challenges and unlock the full potential of AI. This collaborative approach not only enhances our understanding of AI systems but also paves the way for responsible and impactful AI applications.