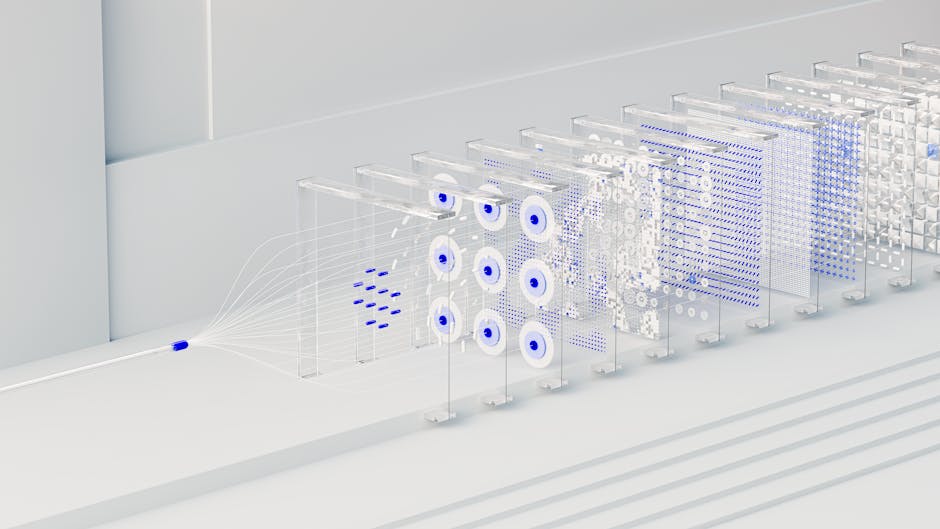
Deep Learning for Fraud Detection
Deep Learning for Fraud Detection
Fraud is a pervasive problem in today's digital world, costing businesses billions of dollars each year. Traditional methods of fraud detection, such as rule-based systems and manual reviews, are often inadequate in detecting sophisticated and constantly evolving fraud techniques.
However, with the advancements in deep learning technology, businesses now have a powerful tool to combat fraud. Deep learning is a subfield of machine learning that focuses on training artificial neural networks to automatically learn and make predictions from large amounts of data.
Deep learning algorithms can analyze vast quantities of transactional and behavioral data to identify patterns and anomalies indicative of fraudulent activity. By processing data in different layers of artificial neural networks, these algorithms can capture complex relationships and uncover subtle patterns that may go unnoticed by traditional fraud detection methods.
One popular application of deep learning in fraud detection is anomaly detection. Anomaly detection algorithms can learn normal behavior patterns from historical data and flag any deviations from the norm as potential fraud. This allows businesses to quickly detect and respond to fraudulent activities in real-time.
Another application of deep learning is in the area of identity verification. Artificial neural networks can analyze multiple data points, such as user behavior, device details, and biometric information, to verify the authenticity of an individual's identity. This approach adds an extra layer of security by reducing the risk of identity theft and account takeover.
Deep learning models are also capable of adapting and improving over time. As more data is collected and processed, the models can continuously learn and update their predictions, making them even more accurate and effective in detecting fraud.
However, it's important to note that deep learning is not a magic solution for fraud detection. It requires large labeled datasets, powerful computation resources, and expert knowledge to train and fine-tune the models. Additionally, deep learning algorithms may occasionally generate false positives or false negatives, requiring human intervention and validation.
In conclusion, deep learning holds great promise for fraud detection and prevention. By leveraging the power of artificial neural networks, businesses can enhance their existing fraud detection systems and stay one step ahead of fraudsters. With continued advancements in technology and an increasing availability of data, deep learning is expected to play a crucial role in the ongoing battle against fraud.